OCR Bivariate Data | Success Guide Part 1
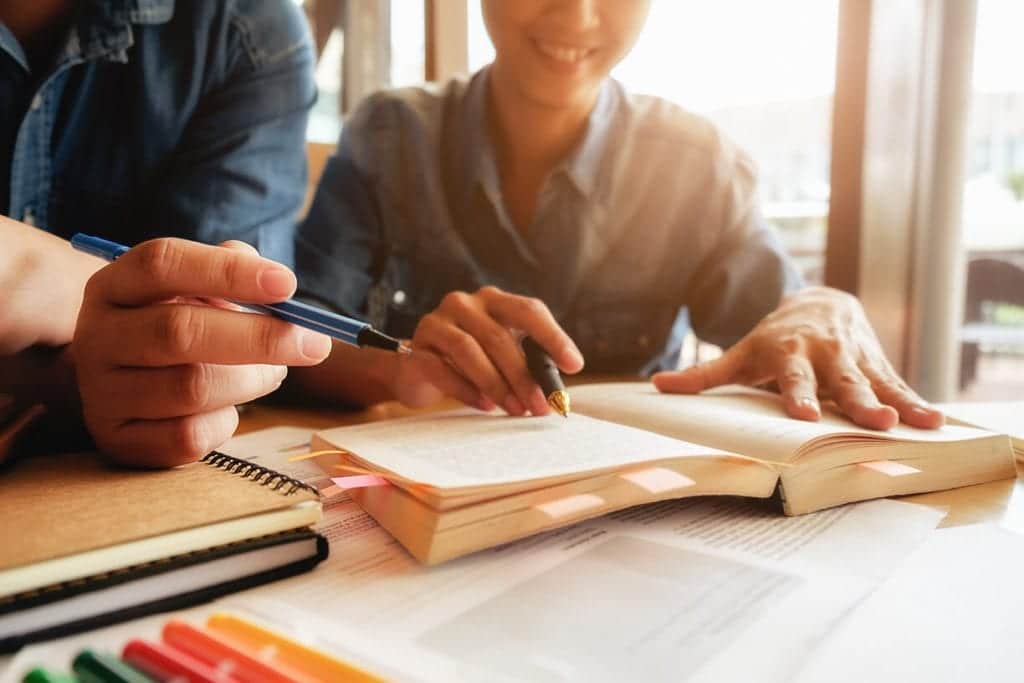
Introduction
You were first introduced to Bivariate data at GCSE! It just wasn’t called that. You would have known it as “scatter graphs” where you looked at the relationship between two variables. Let’s say the temperature on a hot sunny day and the number of people wearing sunglasses.
If you were to gather such data and plot it you would see a positive correlation.
Similarly if you looked at the age of a car and its value, you would notice, in the majority of cases, a negative correlation. We say the majority of cases, because there are a very small minority of cars whose value does appreciate over time.
You will also be introduced to the theory behind the PMCC or Product Moment Correlation Coefficient. This essentially measures the strength of the correlation.
Bivariate Data
The following table shows the test results for 10 students who took a test in maths and art. The results are in percentages.
Maths | Art |
15 | 87 |
35 | 67 |
95 | 20 |
46 | 42 |
78 | 29 |
5 | 98 |
29 | 60 |
75 | 45 |
62 | 52 |
20 | 76 |
From this data is there any type of link between the test results?
An answer can be obtained by drawing a scatter graph with Maths on the horizontal axis and Art on the vertical axis:
Having produced a scatter graph can you see any link between these scores?
Well a high score in maths results in a low score in art and a high score in art results in a low score in maths.
This is not 100% for each instance but you can see that this is the general trend. You can therefore say that there is a negative correlation between these results.
The data in the table depends on a set of pairs of values and this is an example of bivariate data, where each item requires the values from two variables.
Bivariate Data - Random and non-random variables
In the example the results for maths and art have unpredictable values and so they are random. This means that the results are free to assume a set of discrete data within a given range.
There are instances where some results are controlled such as the times when a temperature is taken. Situations where the variable is controlled results in non-random variables.

Bivariate Data – Understanding scatter diagrams
It is possible to determine a correlation by looking at the actual scatter diagram.
Bivariate Data – Product Moment Correlation
The table below shows the results of 10 students who took a test in maths and physics. From the table below can you see a correlation?
\begin{array}{|c|c|c|} \hline \text { Student } & \text { Maths (x) } & \text { Physics (y) } \\ \hline 1 & 31 & 50 \\ \hline 2 & 21 & 24 \\ \hline 3 & 78 & 60 \\ \hline 4 & 75 & 40 \\ \hline 5 & 63 & 59 \\ \hline 6 & 57 & 54 \\ \hline 7 & 13 & 5 \\ \hline 8 & 89 & 78 \\ \hline 9 & 46 & 45 \\ \hline 10 & 57 & 45 \\ \hline \end{array}The mean of the maths test results is:
\bar{x}=\frac{\sum^x}{n}=\frac{530}{10}=53The mean of the physics results is:
\bar{y}=\frac{\Sigma y}{n}=\frac{460}{10}=46A scatter graph is shown with the mean test results highlighted and this suggests a positive correlation.
From this scatter diagram you can see the two highlighted results. One with a better result in Physics compared to Maths and the other with a better result in Maths compared to Physics.
The scatter graph is now shown with two axes that are drawn through the mean point.
The scatter diagram has now been divided into four regions. The mean (\bar{x}, \bar{y}) point is the middle of the diagram and so can be considered as a new origin. Relative to (\bar{x}, \bar{y}) he coordinates of all the other points are of the form \left(x_i-\bar{x}, y_i-\bar{y}\right)
In the regions 1 and 3 the product of \left(x_i-\bar{x}\right)\left(y_i-\bar{y}\right) will be positive for all points.
In regions 2 and 4 the product of \left(x_i-\bar{x}\right)\left(y_i-\bar{y}\right) will be negative for all points.
When there is a positive correlation most of the data points fall into regions 1 and 3. The sum of these terms is denoted by S_{x y}
S_{x y}=\sum_{i=1}^n\left(x_i-\bar{x}\right)\left(y_i-\bar{y}\right)When the correlation is positive you should expect the sum of the terms to be positive and large. When the correlation is negative you should expect the sum of these terms to be negative and large. When there is no correlation the points will be scattered around all regions and in most cases any positive and negative values will cancel each other out.
Pearson’s product moment correlation coefficient
In order to allow for both the number of items and the spread within the data, the value of S_{x y} is divided by the square root of the product of S_{x x} and S_{y y}
The sample product moment correlation coefficient is represented by r and is given by:
r=\frac{\sum\left(x_i-\bar{x}\right)\left(y_i-\bar{y}\right)}{\sqrt{\sum\left(x_i-\bar{x}\right)^2\left(y_i-\bar{y}\right)^2}}=\frac{S_{x y}}{\sqrt{S_{x x} S_{y y}}}The value of r has a value always between +1 to -1.
Example
From a selection of plants which produce carrots only a few plants produce large ones. 5 plants are selected at random and the largest one is weighed.
\begin{array}{|l|c|c|c|c|c|} \hline \begin{array}{l} \text { Number of } \\ \text { carrots, } x \end{array} & 5 & 5 & 7 & 8 & 10 \\ \hline \begin{array}{l} \text { Weight of } \\ \text { largest, } y \\ \text { (grams) } \end{array} & 240 & 232 & 227 & 222 & 215 \\ \hline \end{array}Calculate the sample product moment correlation coefficient.
Solution
n=5, \bar{x}=7, \bar{y}=227.2\begin{array}{|c|c|c|c|c|c|c|} \hline x & y & x-\bar{x} & y-\bar{y} & (x-\bar{x})^2 & (y-\bar{y})^2 & (x-\bar{x})(y-\bar{y}) \\ \hline 5 & 240 & -2 & 12.8 & 4 & 163.84 & -25.6 \\ \hline 5 & 232 & -2 & 4.8 & 4 & 23.04 & -9.6 \\ \hline 7 & 227 & 0 & -0.2 & 0 & 0.04 & 0 \\ \hline 8 & 222 & 1 & -5.2 & 1 & 27.04 & -5.2 \\ \hline 10 & 215 & 3 & -12.2 & 9 & 148.84 & -36.6 \\ \hline 35 & 1136 & \mathbf{0} & \mathbf{0} & \mathbf{1 8} & \mathbf{3 6 2 . 8 0} & \mathbf{- 7 7 . 0} \\ \hline \end{array}\begin{gathered} S_{x x}=\sum(x-\bar{x})^2=18 ; S_{y y}=\sum(y-\bar{y})^2=362.8 ; S_{x y}=\sum(x-\bar{x})(y-\bar{y})=-77 \\ \therefore r=\frac{\sum\left(x_i-\bar{x}\right)\left(y_i-\bar{y}\right)}{\sqrt{\sum\left(x_i-\bar{x}\right)^2\left(y_i-\bar{y}\right)^2}}=\frac{S_{x y}}{\sqrt{S_{x x} S_{y y}}} \\ =\frac{-77}{\sqrt{18 \times 362.8}}=-0.953 \end{gathered}In literally every single instance you can think of, the PMCC can be determined on your calculator. The above is just to explain the theory of where the PMCC calculation comes from.
What you need to know is that:
Correlation of 1 is known as perfect positive correlation
Correlation of -1 is known as perfect negative correlation
Remember that all that these values are showing is the strength of the relationship between two variables.
Statistics is not always the most straightforward area in A Level Maths which is why we have a number of classroom based revision courses that take place during the half term holidays throughout an academic year. We also have pre-exam courses which are more exam paper specific and could be just on A Level Pure Maths, A Level Mechanics or just A Level Statistics.
Once more, all our Maths A Level Revision Courses are 100% bespoke depending on student needs. We do not go over material that students are happy with. The aim is for students to consolidate any gaps in their knowledge.